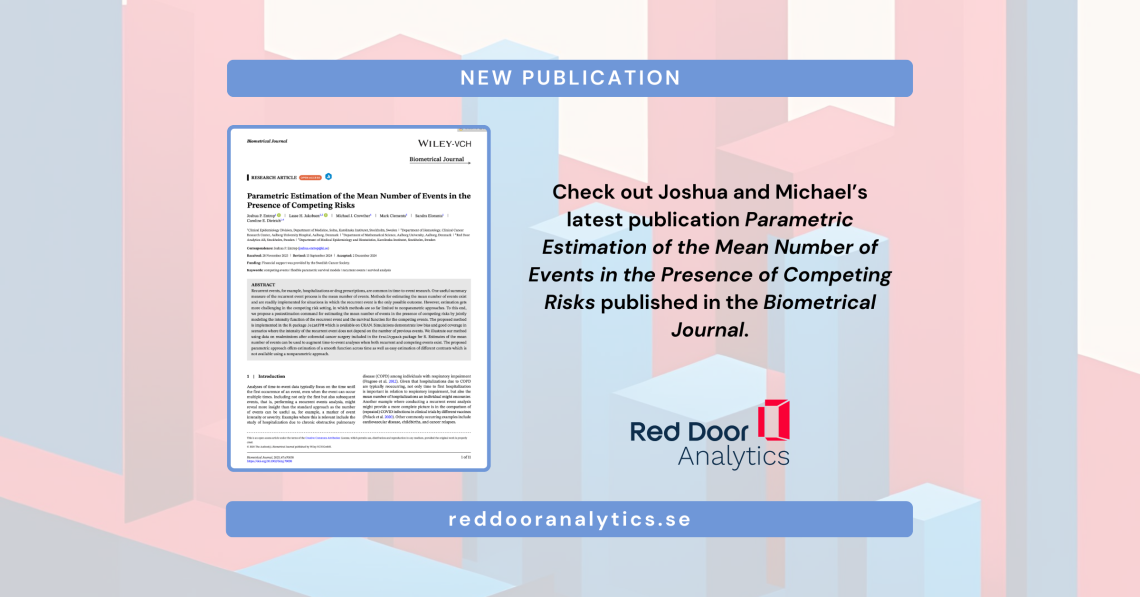
In our latest publication, we introduce a novel parametric model for estimating the mean number of events in the presence of competing risks. This paper was led by Dr Joshua Entrop as part of his PhD project at Karolinska Institutet and co-authored by Dr Michael Crowther. This advanced model simultaneously models both recurrent and competing event processes, enabling us to derive estimates of the average number of events at various time points. Our manuscript has been published open access in the renowned Biometrical Journal.
Also, check out Joshua’s article on reproductive patterns among non-Hodgkin lymphoma survivors, where this method was applied to estimate the average number of childbirths among survivors of non-Hodgkin lymphoma.
Three Questions to Joshua
In which projects can this method be applied?
Estimates of the mean number of events provide a valuable summary measure for recurrent event processes in the presence of competing risks. A recurrent event refers to an event that can occur multiple times for the same individual, which is common in medical research. Examples include infections, cancer recurrences, hospitalizations, or childbirth. However, individuals may face in addition to the recurrent event also competing events, such as death, which may prevent them from experiencing the recurrent event, which complicates the estimation.
Our new model addresses this challenge of modelling both the recurrent event and the competing event process simultaneously. It provides a smooth estimation of the average number of events over time, offering an easily interpretable summary measure. For instance, we can estimate the average number of hospitalisations after colon cancer surgery, which could offer valuable insights into recovery patterns.
What are the advantages of using this method compared to standard time-to-event models?
Traditional time-to-event analysis often focuses on modelling the time to the first event occurrence. This approach is suitable for events where the individual enters a stable, irreversible state, such as a chronic condition like diabetes. However, for recurrent events, where multiple occurrences are possible, understanding the frequency and timing of these events can provide important additional information about disease progression or recovery.
For example, estimating the mean number of hospitalizations following colon cancer surgery can serve as an indicator of recovery. Patients with more hospitalisations likely face more severe complications, suggesting a slower or more complicated recovery compared to those with fewer hospitalisations.
What are the limitations of this model?
While our model provides a useful and interpretable summary of the recurrent event process, it is, by nature, a summary measure. For more detailed analysis of the recurrent event process, other modelling approaches may be more suitable. Multi-state models, for instance, can explicitly model transitions between different states or event occurrences, offering deeper insights into the underlying processes. For example, multi-state models can help identify risk factors influencing specifically whether a patient will experience a third or fourth hospitalisation, offering more granular understanding.
Both recurrent event analysis and multi-state models are specialist areas at Red Door Analytics. If you’re considering a project that could benefit from these methods, don’t hesitate to contact us. Also, don’t forget to sign up for our newsletter or follow us on LinkedIn to stay updated on our latest offerings and courses, e.g., on multi-state models.
Publications
- Entrop JP, Jakobsen LH, Crowther MJ, Clements M, Eloranta S, Dietrich CE. Parametric estimation of the mean number of events in the presence of competing risks. Biometrical Journal 2025; 67(1): e70038
- Entrop JP, Weibull CE, Smedby KE, Jakobsen LH, Øvlisen AK, Glimelius I, Marklund A, Larsen TS, Holte H, Fosså A, Smeland KB, El-Galaly TC, Eloranta S. Reproduction patterns among non-Hodgkin lymphoma survivors by subtype in Sweden, Denmark and Norway: A population-based matched cohort study. British Journal ofHaematology 2023; 202(4): p 785-795